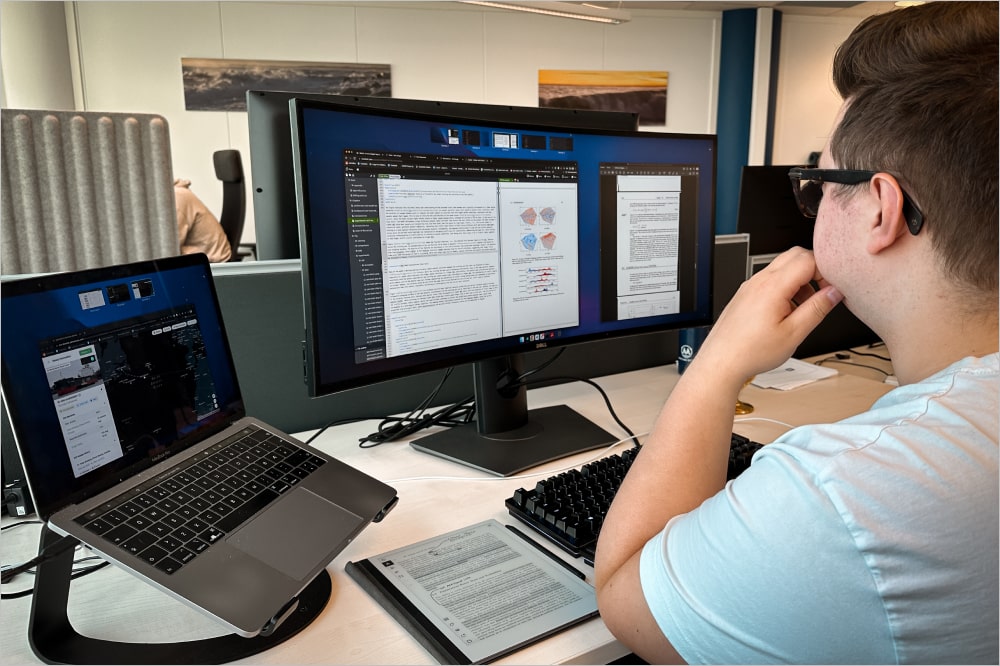
Interview - Jørgen's Master Thesis
What is your relation to Maritime Optima?
My journey with Maritime Optima started when the company was in its early stages. I joined as one of the first full-stack developers and laid much of the initial frontend and backend foundation we have built upon today. As the team expanded with backend developers, my focus gradually shifted towards the frontend and product design. Additionally, I have always been fascinated by AI, and so I began my Artificial Intelligence (AI) studies at the Norwegian University of Science and Technology in 2020. Despite academic commitments, I remained tightly connected to Maritime Optima, contributing as much as possible. My involvement ranged from rebuilding our mobile application from the ground up to taking on leadership roles in designing and developing several key features. Maritime Optima also gave me an excellent opportunity to apply the theoretical knowledge I gained in my AI studies, using our data and real-life use cases.
What is the name of your thesis, and what is the scope of it?
The title of my thesis is "Expanding Our Knowledge of Maritime Trade with AIS and Explainable AI Systems". It is an interdisciplinary study that intertwines AI and maritime trade analytics. The thesis delves into the development and interpretation of AIS-based machine-learning (ML) forecasting models, with a particular emphasis on demystifying their decision-making processes when predicting financial instruments in the maritime industry.
What was the motivation behind your Master's thesis?
Early on, we noticed that the ML models were quite proficient at forecasting financial instruments based solely on AIS data. This sparked our curiosity, and we naturally wondered why and how these AI models achieved such remarkable results. Further, as we have seen through AI systems such as AlphaZero, when these models exhibit decision-making capabilities that match and sometimes even outperform human understanding, it presents a compelling opportunity for us to learn from these systems. However, modern models are exceedingly complex, with their problem-solving abilities growing parallel with their complexity, making it progressively more challenging to comprehend their rationale. Hence, the primary motivations behind my thesis were grounded in the underlying performances of the models, as well as the drive to reveal the decision-making process behind them. This would not only build a promising foundation for future forecasting models but also bring more transparency into the industry.
What has been done by previous students and companies within related topics?
Market forecasting often falls into the "behind closed doors" domain, which makes it likely that many projects in this area remain under the radar. Despite this, a limited yet valuable body of academic research explores forecasting financial instruments and freight rates using AIS data in combination with other macroeconomic variables. These studies have found that incorporating AIS data improves the predictive capabilities of their models, a finding that greatly encouraged my thesis work. However, these studies primarily concentrated on the results generated by these models, leaving the decision-making processes of the models, the steps they take, and the factors they consider when making forecasts largely uncharted. Additionally, these studies typically employ relatively simple aggregations of AIS variables in larger ocean areas, which does not necessarily provide a detailed explanatory medium to derive new knowledge. This intriguing gap, particularly with the potential for more complex AIS features, opened a unique research opportunity.
How did you approach the problem?
I was fortunate to have a team of skilled maritime professionals from Maritime Optima supporting and guiding me. Their advice and knowledge of the maritime industry enriched my understanding of the problem, which naturally split into two main research objectives:
- Creating a sufficient AIS-based forecasting model
- Derive insight into its decision-making process and assess this together with Maritime Optima professionals.
My research method consisted of a literature review of the current academic landscape to find relevant state-of-the-art models and explanation methods, which were then materialized. The models were run through several experiments to find the optimal one, where the experiments saw the models forecast various relevant maritime financial instruments. Through explanations based on SHAP, we assessed and evaluated the decision-making of the best-performing model for each experiment and instrument.
What was the biggest challenge?
The biggest challenge of the thesis was finding an adequate explanation medium for the models - considering the multidimensional nature of the data, stretching over both time and a complex set of AIS-derived variables. Also, these explanations had to be understood by non-technical maritime professionals unfamiliar with the complex models' inner workings. In the end, the model’s explanations ended up being of an ambiguous nature, making it more challenging to make concrete conclusions about the AIS and its interplay with the market.
What have you learned? And how will you use that knowledge in your future work?
Throughout my thesis journey, I have learned a lot about both the complexities of maritime trade and the potential of artificial intelligence. It became clear that data-driven analysis will play a huge part in understanding this industry, and AIS data has a lot more potential than we might initially think, especially in creating forecasts. Also, diving into explainable AI was quite an eye-opener. It's crucial to not only develop a model that makes the right predictions but also understand why those predictions are made. This transparency builds trust in AI systems and can lead to exciting new insights.
Looking to the future, I'm eager to apply what I've learned. The understanding I've gained about maritime trade will definitely shape how I approach industry problems. Also, having worked extensively with AIS data and machine learning models, I'm excited to see how we can continue to use this resource to its full potential. Working on this thesis allowed me to understand various ML models and XAI techniques better, which has prepared me well for future challenges. I'm looking forward to using these skills in my future work.
Do you feel like your scholarship from Maritime Optima has helped when writing your Master's thesis, and in what way?
Without a doubt - the scholarship from Maritime Optima played a crucial part in the success of my Master's thesis. The scholarship made it easier for me to study remotely from Oslo, near Maritime Optima's offices. This location advantage facilitated easier and more effective communication with the company, which was invaluable during the course of my research. The financial backing provided by the scholarship was particularly helpful in mitigating any potential technical constraints. It allowed me to access advanced computational resources and virtual machines, significantly speeding up model training and research experimentation. This not only boosted the efficiency of my work but also allowed me to explore broader and more complex problem spaces.
Would you recommend this scholarship to other students?
I highly recommend this scholarship to other students. The unique blend of academic and practical opportunities it provides is exceptional. The scholarship not only offers financial support but also facilitates an immersive industry experience. Working with Maritime Optima, students gain firsthand experience and insights into the maritime industry, which enhances their understanding and perspective on the subject matter. It is an excellent platform for students who wish to apply their academic knowledge to real-world problems and gain valuable work experience. Moreover, Maritime Optima's collaborative and supportive environment ensures that students have all the resources and guidance necessary to succeed in their research endeavors.